In today’s digital age, data drives decision-making processes across various industries. As we delve into the vast realms of consumer behavior data, it’s crucial to understand that not all data is created equal. Anomalies, or irregularities, often creep into datasets, distorting the accuracy and reliability of the information they provide. Here’s a brief description of the kinds of anomalies we’ve encountered, with some detail about how Azira goes about identifying and eliminating them, ensuring the integrity of the consumer behavior data we share with our customers.
Understanding Data Anomalies
At Azira, we gather data from diverse sources, offering valuable insights into consumer behavior. However, the ever-evolving mobile ecosystem brings with it an influx of anomalous data that can misrepresent real consumer actions. Some common anomalies we encounter include:
device histories.
patterns (such as high traffic at a store that closed).
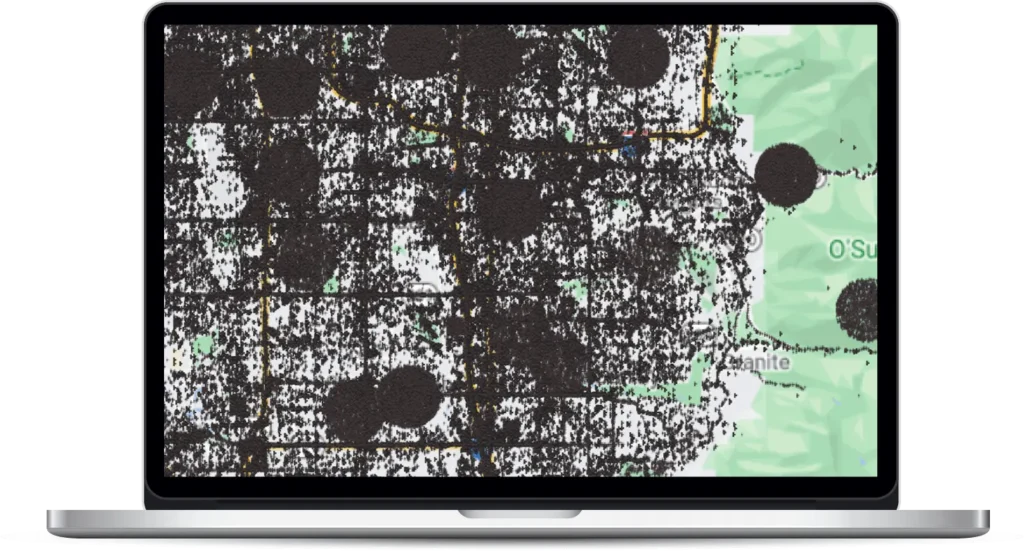
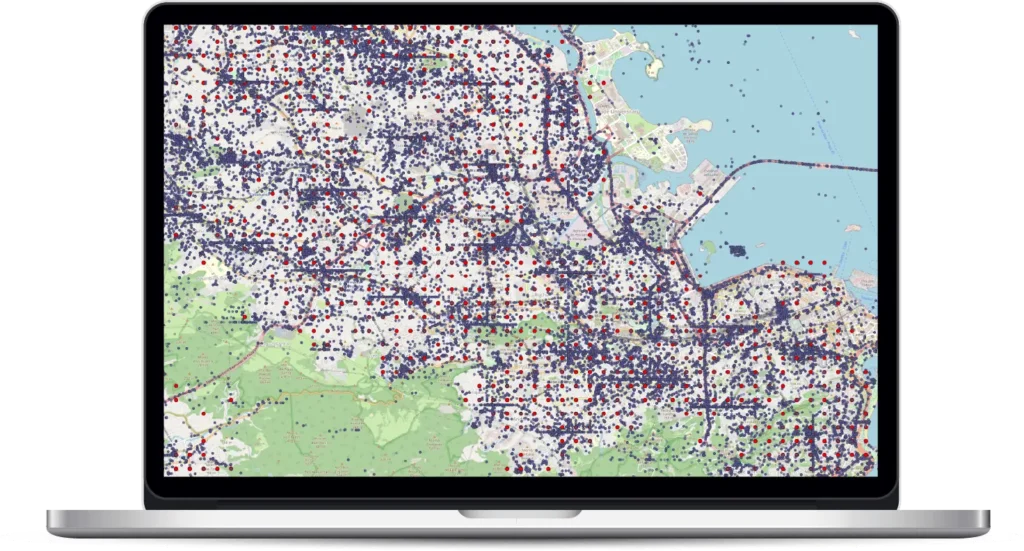
Azira’s Data Anomaly Filters
Azira has developed sophisticated algorithms that incorporate image recognition and advanced statistical analyses to detect and remove these anomalies. Additionally, we are continuing to research and develop new AI-based methodologies. However, as human movement, mobile device usage, and the fragmented mobile data ecosystem continue to evolve, we face a variety of challenges:
Emergence of New Anomalies: Constant vigilance is required as new anomalies and fraudulent data sources regularly appear.
Balancing Data Volume: Aggressively removing anomalies can impact data volume. We aim for a balanced approach to ensure accurate data points are not discarded via overzealous scrubbing.
Computational Complexity: Identifying complex anomalies at scale can be computationally intensive, demanding more computing resources and advanced techniques like image recognition and statistical analyses.
A Commitment to Data Quality
In the face of these challenges, Azira remains steadfast in its commitment to maintaining high data quality standards. Our ongoing research and development efforts focus on innovative methodologies, incorporating cutting-edge technologies to safeguard the value of our data. As we continue to evolve alongside the dynamic mobile data landscape, Azira persistently explores new avenues, ensuring the data you rely on is accurate, reliable, and free from anomalies.
For our latest anomaly updates, please review our topical data sheet, Understanding Data Anomalies in Consumer Behavior Data.